Tags: Adaptive Learning, course content, Differentiated Instruction, Learning Paths, Mastery, MasteryPaths, Peer-Reviewed Entry, Personalized Learning
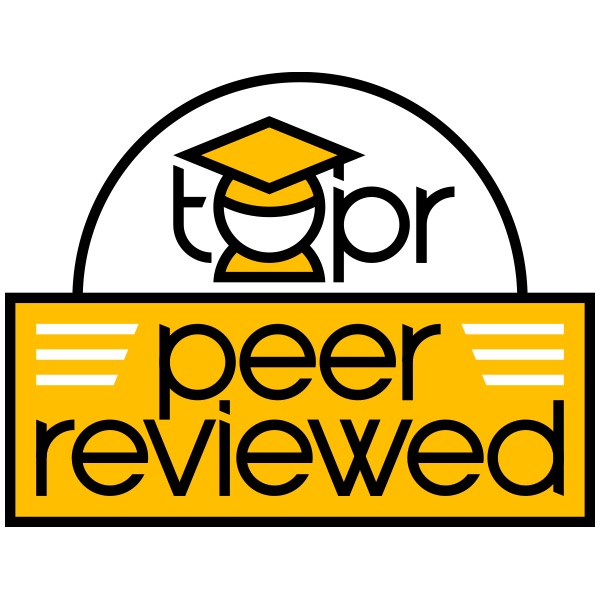
Description
Background
Learning content is often presented to students in a variety of ways throughout an online or blended course, but this doesn’t necessarily always translate into them doing equally well on their assignments and assessments (Kizilcec & Halawa, 2015). For example, some students may skip a required reading, but watch a video, or may simply go directly to work on an assignment and only refer to the learning materials as needed. This has, at times, led to less-than-ideal assignment and/or assessment performance, which is evidence that a knowledge gap exists in the student’s understanding of a given topic. In order to mitigate the negative effects of this type of learning behavior, faculty members might turn to a feature like Canvas MasteryPaths to help personalize the student learning experience through frequent (ungraded) diagnostic tests and targeted content scaffolding based on students’ learning performance, until learning mastery (Bloom, 1984; Essa & Laster, 2017) is achieved.
Design Considerations
To design a personalized learning experience using MasteryPaths, it is important to begin by thinking of the summative assignment / assessment; this way you will have an idea of your targeted outcome(s). Then after determining what students will do to show mastery, you can then begin to think about which components will be part of the core content you want all students to see, and which content will only be supplied to students who require additional scaffolding.
The ‘single-phase’ design referenced in this write-up, will conform with the following schematic. This schematic can be accessed, downloaded, and edited under an open license (CC BY 4.0) via diagram.net. (cf. Figure 1 linked text)
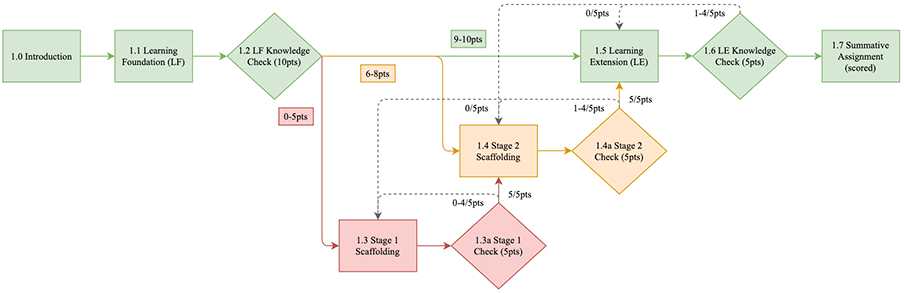
Below is the instructor view of the same ‘single-phase’ design configuration in Canvas. This template can be accessed freely under an open license (CC BY-SA 4.0) in the Canvas Commons: https://tinyurl.com/xukhjere.
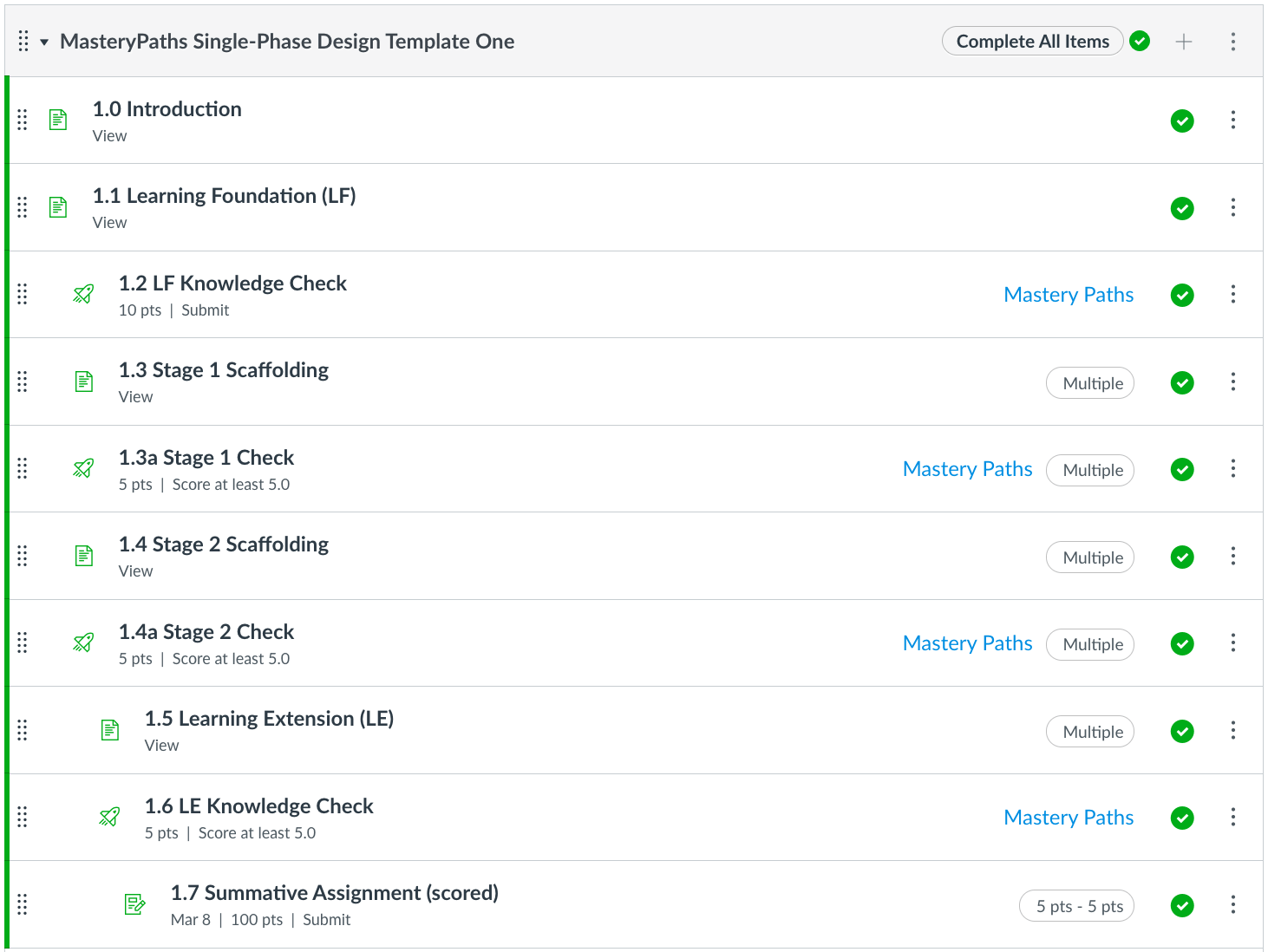
While you can utilize any scored assignment type in Canvas, you may find that using (auto-graded) multiple-choice quizzes provides students the most seamless experience, as they won’t have to wait around for you to grade an assignment or discussion post. That is to say, be sensitive to the frustration students might feel due to the additional work being added to their learning journey. It is helpful to estimate around double the time you think students will have to spend to go through every step.
In terms of guiding and measuring students through their personalized journey, it is recommended to make the initial Knowledge Check (which determines their starting point) and every part of the formative learning beyond that (through MasteryPaths) to be ungraded, as not to make this experience punitive while students work to grasp certain concepts. Your final assignment / assessment, which will be scored and recorded in the gradebook, should be adequate in determining their ability levels.
Finally, after mapping out your plan, you will have to consider the technical affordances Canvas provides through the MasteryPaths feature. There are limitations to the number of pathways (3) per scored item, so if you require a more dynamically mapped experience, you may decide to add multiple phases to the scaffolding process. (Keep in mind, however, the level of ‘forced’ participation you are requiring from students—particularly those who might struggle—in a multi-phase design. This topic will be discussed in more detail in a separate article.)
Development Notes
You are now ready to develop the content to help execute your design goals. Remember that a key component of designing meaningful personalized learning experiences for students is keeping your learning content granular enough to be encoded (info in), stored (info sticks), and ultimately retrieved (info out) under reasonable conditions, as determined by cognitive theories of information processing and remembering (de Bruin, & van Merriënboer, 2017; Fountain, & Doyle, 2012).
Link to example artifact(s)
Assembly Notes
In the MasteryPaths single-phase design template (cf. Figure 1), you begin to discern how the learning experience requires varying levels of content development for each discrete section.
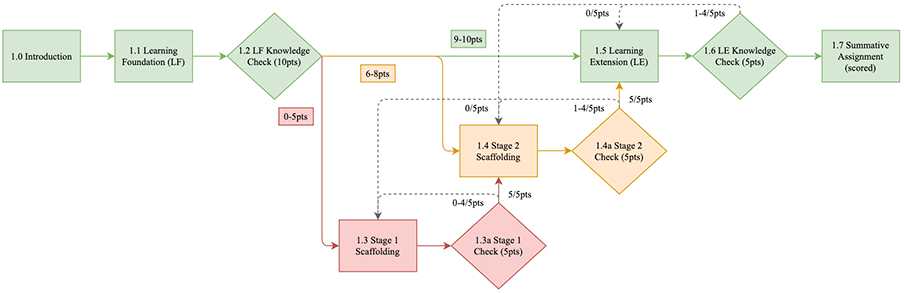
The Introduction [1.0] explains how the personalized, mastery-based ‘experience’ works, as students may not be accustomed to the MasteryPaths user-experience. Next, the Learning Foundation (LF) [1.1] and the corresponding LF Knowledge Check (KC) [1.2] serve as a basis for the learning content along with a pre-test of sorts to determine if students are equipped to proceed to the Learning Extension (LE) [1.5].
The LF KC should have pools of questions that correspond to the ‘stages’ of the learning experience: three (3) questions from Stage 1, four (4) questions from Stage 2, and three (3) questions representative of the LE. (This distribution helps ensure students exhibit sufficient general competence before proceeding.)
Note: The LF should be comprised of the core knowledge required to complete the Summative Assignment (SA) [1.7]—content that if not mastered would provide a considerable barrier to meeting the learning outcome(s).
While the LF provides the base knowledge required to succeed on the SA, the LE and LE Knowledge Check (KC) are also key components for students—often involving some level of higher-order thinking about the topic. An example of this might be learning how to use a foreign language in an authentic context (i.e., LE 1.5), while also being able to perform the basic function of constructing grammatically correct sentences (i.e., LF 1.1).
Making the leap from LF (low-level Bloom) to LE (mid-to-high level Bloom) is where this model predicts students may start to most profoundly deviate from each other in terms of learning trajectories; hence, two stages of scaffolding are designed to catch students who are not quite ready to make the full jump from LF to LE. To illustrate, Stage 1 Scaffolding (S1) [1.3] and Stage 1 Check (C) [1.3a] are situated to support students who are struggling the most, while the Stage 2 Scaffolding (S2) [1.4] and Stage 2 Check (C) [1.4a] are meant to build on S1 and/or provide a moderate level of support for those who performed averagely or slightly above average on the LF KC.
All the aforementioned lead to the SA, which is the only scored item in the sequence that gets recorded in the gradebook. The idea is that by the time students reach this point—if the learning content, KCs, and Cs were thoughtfully designed to support subject-area mastery—students should be equipped to move through the SA confidently and successfully.
Lastly, create a plan to collect data produced from this educational endeavor and determine a sound method by which to analyze it so you can continue to make iterative improvements on your model and the content therewithin.
Implementation Status
This template is currently being piloted in the departments of Political Science and Modern Languages, and while the content within each component and titles given may vary across disciplines, the general principles of the single-phase design has been consistently well-received, and as data is collected on the impact this instructional model has on student success, it will continue to undergo iterative improvements and be shared back out with the educational community.
Link to scholarly reference(s)
Bloom, B. (1984). The 2 Sigma problem: The search for methods of group instruction as effective as one-to-one tutoring. Educational Researcher, 13(6), 4–16. https://journals.sagepub.com/doi/pdf/10.3102/0013189X013006004
de Bruin, A. B. H., & van Merriënboer, J. J. G. (2017). Bridging cognitive load and self-regulated learning research: A complementary approach to contemporary issues in educational research. Learning and Instruction, 51, 1–9. https://doi.org/10.1016/j.learninstruc.2017.06.001
Essa, A., & Laster, S. (2017). Bloom’s 2 Sigma problem and data-driven approaches for improving student success. The First Year of College: Research, Theory, and Practice on Improving the Student Experience and Increasing Retention. https://doi.org/10.1017/9781316811764.009
Fountain, S. B., & Doyle, K. E. (2012). Learning by Chunking. In N. M. Seel (Ed.), Encyclopedia of the Sciences of Learning (pp. 1814–1817). Springer US. https://doi.org/10.1007/978-1-4419-1428-6_1042
Kizilcec, R. F., & Halawa, S. (2015). Attrition and Achievement Gaps in Online Learning. Proceedings of the Second (2015) ACM Conference on Learning @ Scale, 57–66. https://doi.org/10.1145/2724660.2724680
Citation
Paradiso, J., & Chen, B. (2021). Personalized learning design with canvas masterypaths. In A. deNoyelles, A. Albrecht, S. Bauer, & S. Wyatt (Eds.), Teaching Online Pedagogical Repository. Orlando, FL: University of Central Florida Center for Distributed Learning. https://topr.online.ucf.edu/personalized-learning-design-with-canvas-masterypaths/.Post Revisions:
- July 13, 2021 @ 17:50:12 [Current Revision]
- July 13, 2021 @ 17:50:12
- July 13, 2021 @ 17:47:12
- July 13, 2021 @ 12:16:00
- July 12, 2021 @ 10:58:23
- July 12, 2021 @ 10:57:40
- July 6, 2021 @ 13:42:26
- July 6, 2021 @ 13:41:10